We would all like to fancy ourselves as eminently capable of impartiality, able to make decisions without prejudices – especially at work. Unfortunately, the reality is that human bias, both conscious and unconscious, can’t help but come into play when it comes to who gets jobs and how much money candidates get offered.
Managers often gravitate to people most like themselves, make gender-based assumptions about skills or salaries, or reject candidates who have non-white names – to name just a few examples – even if they don’t mean to.
There’s an increasingly popular solution to this problem: why not let an intelligent algorithm make hiring decisions for you? Surely, the thinking goes, a computer is more able to be impartial than a person, and can simply look at the relevant data vectors to select the most qualified people from a heap of applications, removing human bias and making the process more efficient to boot.
A wealth of startups and associated technology tools have sprung up in recent years to address the appetite for more diverse workforces. The Gapjumpers platform promises “blind audition” technology where “gender, education and background don’t matter” to the quest to find top talent. Entelo’s recruitment software has been billed as able to “get more women hired”, while Doxa helps you “find tech companies where female employees thrive. From HireVue and Gild toTextio, Jobaline and Korn Ferry, there are no shortage of headhunting and recruitment firms turning to the “magic” of algorithms to make attracting and hiring the right people more efficient and more effective – all while theoretically casting a wider net to draw candidates who might get left out by traditional “gut instinct” methods.
But there’s an unaddressed issue here: any algorithm can – and often does – simply reproduce the biases inherent in its creator, in the data it’s using, or in society at large. For example, Google is more likely to advertise executive-level salaried positions to search engine users if it thinks the user is male, according to a Carnegie Mellon study. While Harvard researchers found that ads about arrest records were much more likely to appear alongside searches for names thought to belong to a black person versus a white person.
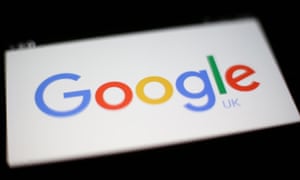
These aren’t necessarily malicious situations – it’s not that Google is staffed by sexists, for example, but rather that the algorithm is just mirroring the existing gender pay gap. But in so doing, the algorithm reinforces that gap, and as long as we continue to believe an algorithm is an “unbiased” machine, we risk reinforcing the status quo in harmful ways. When bias appears in data, it even seems to suggest that historically disadvantaged groups actually deserve the less favourable treatment they receive.
While algorithms might work with data alone, it’s always human beings that decide what factors they weigh. Law professor and sociologist Ifeoma Ajunwa is authoring a paper on hiring by algorithm, and she asserts that many of the data points we think of as “neutral” – housing status, education level, credit score or even criminal record – are actually wrapped up in assumptions that ignore elements of racial inequality. She notes this “societal noise” plays a role in reinforcing our assumptions about data: for example, we may view a standardised test score as a fair measure of aptitude, but we rarely ask how those scores function in communities where schools are racially and economically segregated. When not all students begin at the same level of access to resources, a test score offers an incomplete picture.
“While seemingly innocuous or even meritocratic, educational pedigree strongly correlates to both class and race,” Ajunwa tells me. “Educational pedigree, in several instances, may be ‘societal noise’ in regards to fit for the job, as the school an applicant attended may not accurately predict fitness or skill set for a specific role.”
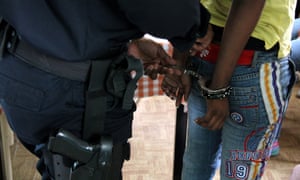
Complicating the discussion on bias in algorithms is the fact that companies’ tech is so often closely guarded as a trade secret – without access to the tech itself, it’s tough for an outside party to really test a hiring algorithm’s supposed fairness. But Ajunwa says it’s possible to determine that an algorithm may discriminate based on what types of data it uses to vet candidates and what kind of decision it makes as a result. To use a blunt example, when incarceration policies or the environment of state violence in America both affect black men to a disproportionate degree, an algorithm that automatically delivers a “don’t interview” verdict to candidates with past criminal records therefore disproportionately impacts black job seekers.
“Given the mass incarceration crisis [in America], a salient factor is incarceration record. Currently, employers with ‘check the box’ policies can summarily eliminate applicants with incarceration records [by using] a hiring algorithm,” says Ajunwa. “Similarly, long periods of unemployment might trigger hiring algorithms to exclude applicants, regardless of the reason for the absence from the workplace, thus negatively impacting veterans [as well as] parents returning to the workplace.”
Ajunwa’s colleagues Sorelle Friedler and Suresh Venkatasubramanian also co-authored a paper proposing a potential fix that cuts through the “societal noise” around hiring algorithms’ data points. Here’s how it could work: if, let’s say, credit scores are predisposed to the advantage of white candidates, then rather than looking for an overall top percentile or certain range of scores among all applicants, the high-end range could be studied separately among each racial or gender group and then collected to create a universal average.
“Each feature in the data set is considered separately,” Friedler explains. “For each feature, the per-group scores are considered and modified so that, taken as a whole, someone’s [membership in a historically disadvantaged group] can no longer be inferred from this feature by any algorithm. We do this modification delicately, so that other useful information is not destroyed.”
Rather than having an algorithm count, say, the top 10 percent of all applicant test scores, you could take the top 5% of men’s scores and the top 5% of women’s scores, group them together and then derive a median that applies to all candidates. Although this would require applicants to supply their gender and race on applications for the proposed fix to work, such a repair, the researchers claim, would go further than simply correcting a bias toward disadvantaged groups – it would guarantee no disparate impact on any group, a requirement to make the repair legal to perform.
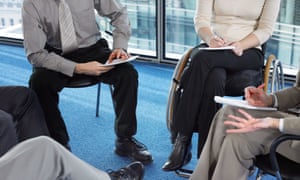
“We present our solution as a discretionary one for employers to adopt and also as a procedure that governmental agencies, such as the EEOC [US federal agency the equal employment opportunity commission], could mandate as an audit for employers who have had complaints logged against them” Ajunwa suggests. “Thus, the repair could serve as both a self-management tool or as an investigatory tool for the government.”
One of the challenges in repairing and perfecting algorithm-based hiring is that there is no standard way to measure the outcome of an algorithm’s choices – how do we know it really is picking the “best” candidates most fairly? How well would an employee have to perform and for how long to have been considered a “correct” or “successful” pick, and how can we evaluate either the appropriateness or the diversity of its recommendations?
“Our work doesn’t answer these questions,” Venkatsubramanian says. “We have to balance the desire for fairness with the desire for effectiveness of prediction, but the assessment of ‘effectiveness’ currently comes from possibly flawed data, such as flawed employee performance assessments.”
The work of these researchers points to a problem in the world of big data that doesn’t get discussed often enough: unless the data itself can be truly said to be “fair”, an algorithm can’t do much more than perpetuate an illusion of fairness in a world that still scores some people higher than others – no matter how “unbiased” we believe a machine to be.
Ajunwa likens it to the stories of the Greek oracle, distorted through history and pop culture references as some sort of great all-knowing voice – when in mythology oracles actually demanded much intuition, discussion and context to interpret. An algorithm is no oracle, but needs rigorous study and repair if the promises made by all these vast, supposedly tech-savvy recruitment firms are to be met.
[source :-theguardian]